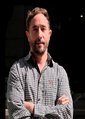
Alvaro Olivera-Nappa
University of Chile, Chile
Title: VHL-Hunter: A predictor of the impact of missense mutations in the function of the Von Hippel-Lindau protein (pVHL), risk of clear cell renal carcinoma and severity of pVHL-associated diseases
Biography
Biography: Alvaro Olivera-Nappa
Abstract
Von Hippel-Lindau (VHL) disease is an autosomal dominant syndrome associated with multiple tumors including hemangioblastoma, clear cell renal carcinoma (ccRCC) and pheochromocytoma (PCC), which results from mutations in the VHL gene. VHL disease is classified into type 1 or type 2 depending on the presence or absence of PCC. A major limitation is that accurate classification can only be made in large kindreds. Furthermore, its use in assisting clinical management is limited since a family may move from one subtype to another. More than 600 VHL disease mutations are missense, which are broadly distributed throughout the gene. Inheritance of VHL mutations in an autosomal recessive fashion can lead to congenital polycythaemias. Germline VHL mutations account for up to 50% of patients with apparently isolated familial PCC and 11% of patients with an apparently sporadic PCC. Additionally, somatic biallelic inactivation of VHL also occurs in the majority of sporadic ccRCCs, with ~250 different missense mutations described. Numerous studies have investigated with conflicting results whether partial/total loss of VHL function or the type of VHL mutation may influence prognosis in ccRCCs. Our group previously developed symphony, a predictor of ccRCC risk associated to VHL mutations. In this work we present VHL-Hunter, a new computational approach to predict all VHL-associated disease risks, which combines structural and H-bond network induced pVHL amino acid partitioning, MOSST and SDM predictions. VHL-Hunter correctly predicts the disease outcome of known pVHL mutations with high accuracy (0.96) and informedness (0.9), and also the outcome of previously undescribed mutations. VHL-Hunter also gives clues regarding the mechanism underlying disease generation and is able to correlate the predicted degree of protein malfunction with the severity of clinical phenotypes, in agreement with experimental data. This method can also be successfully applied to obtain highly accurate predictors of mutation phenotypic outcomes for other proteins